AI Is Just as Biased as We Are
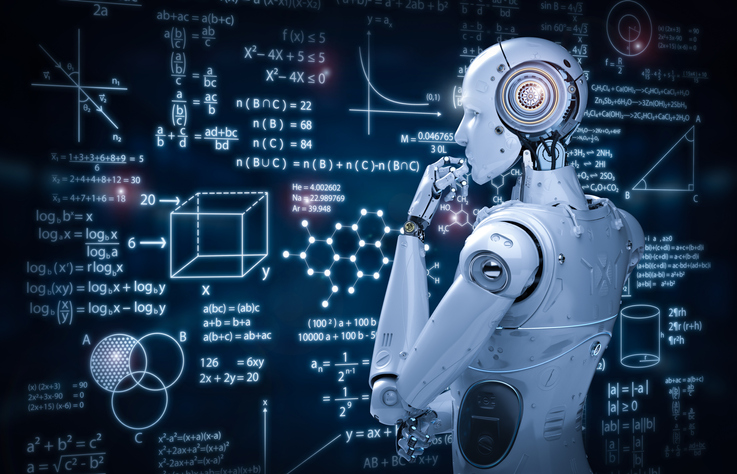
You may have heard: Amazon’s job recruiting tool didn’t like women. The tool was built to review job applicant resumes and identify the most qualified candidates. The issue was that the AI had been trained via patterns observed in resumes submitted over the previous ten years. The vast majority of those resumes came from men. Thus, the AI downgraded resumes that had the word “women’s” in them, as in “women’s studies,” or “women’s volleyball team.” It also downgraded those of women from majority female schools. Amazon says it scrapped the tool after these findings came to light.
It seems that AI is only as “fair” as the historical data fed into it, or the knowledge of the individuals doing the feeding. The ACLU used congressional headshots matched against a database of mugshots to test the accuracy of Amazon’s Rekognition facial recognition software. Despite the fact that only 20% of the congressional photos showed people of color, they comprised 40% of the misidentifications.
You may have read about Amazon’s Ring, a camera-doorbell product. Amazon has partnered with 400 police forces all over the country in an attempt to create an electronic “neighborhood watch.” However, if facial recognition is being used, and people of color are twice as likely to be misidentified, you can imagine the potentially deadly doorstep police confrontations as, guns drawn, cops face down an entirely innocent individual misidentified as a criminal due to the poor quality of facial recognition software.
Even algorithms that seem to target utterly neutral parameters are being fed data fueled by bias. According to the Brooking Institute:
“…evaluations of creditworthiness are determined by factors including employment history and prior access to credit—two areas in which race has a major impact. To take another example, imagine how AI might be used to help a large company set starting salaries for new hires. One of the inputs would certainly be salary history, but given the well-documented concerns regarding the role of sexism in corporate compensation structures, that could import gender bias into the calculations.”
In the healthcare field, algorithms are increasingly used to aid in diagnosis and treatment. However, while 40% of Americans are people of color, 80-90% of clinical trial participants identify as white. Some diseases like diabetes and heart disease that disproportionately impact minorities, but treatments are based on success with non-minority populations. A writer for Wired noted that she had difficulty getting a mammogram referral at 34 because the recommended age is 40, based on data gained from white women. However, black women are typically diagnosed with breast cancer at a younger age than white women.
After having gotten a vitamin D screening that showed a deficiency, I received a letter from my insurance company denying the payment because it was deemed “unnecessary.” African Americans suffer higher rates of vitamin D deficiencies than whites, but the insurance company is clearly basing its guidance on white subjects. Had I sought insurance approval prior to the test, my deficiency would have gone unnoted, and unaddressed.
We have been trained to believe that technology is neutral, that it sidesteps our human biases and preconceptions to leave us with a pure reading of merit or health or employability. However, it’s become clear that technology will not free us from the long, hard work of undoing our biases and preconceptions. If we continue to fail to do that work, our technology will simply entrench and exacerbate societal inequalities.
Leonce Gaiter – VP Content & Strategy